MSU researcher develops imaging-based method of identifying defects in poultry production
Yuzhen Lu, Biosystems and Agricultural Engineering assistant professor, is developing a new imaging-based method of identifying muscle myopathy in poultry production.
In food systems, consumers drive many of the changes producers make in their production practices. This change can be seen in both food and animal production, and poultry production is no exception. Breast meat, also known as white meat, is generally considered healthier and more desirable to consumers. Producers have been selecting birds for larger breasts to get more meat per bird. This meets consumer demands but has come with new challenges.
One of those challenges is the prevalence of muscle myopathy, which is a change in muscle texture that downgrades meat quality. Muscle myopathy such as woody breast and white striping are having a high economic impact on producers. Identifying muscle myopathy has usually been done by skilled personnel in production lines during processing, which is labor-intensive and subjected to human evaluation error. Yuzhen Lu, Ph.D., is a Biosystems and Agricultural Engineering assistant professor and has been developing a new imaging-based method of identifying muscle myopathy that is non-destructive, objective, and amenable to automation. The goal is to maintain a high-quality product, provide quick and accurate identification of the muscle myopathy, while providing cost savings to the poultry processors.
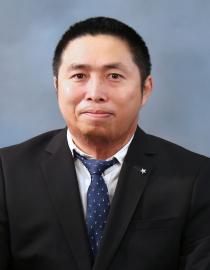
“The poultry processing industry is operating on small margins.” said Lu. “This industry has a notoriously high labor turnover rate. Processors are under mounting pressure to reduce labor dependence and costs while delivering higher quality products, to remain competitive and profitable.”
Lu’s focus is the use of optical imaging technology and deep learning in Artificial Intelligence (AI) systems to help processors identify myopathies and improve quality control. Optical imaging technology uses cameras to look at the meat for defects. The deep learning aspect is when the system takes all the historical knowledge and images given to it by developers to learn to identify muscle myopathy effectively. As the system identifies more defects, it will get faster and more accurate in identification.
“AI models are data-hungry and computationally intensive.” said Lu. “But with dedicated hardware and optimized model architectures, they can be readily used for online meat inspection at video frame rates.”
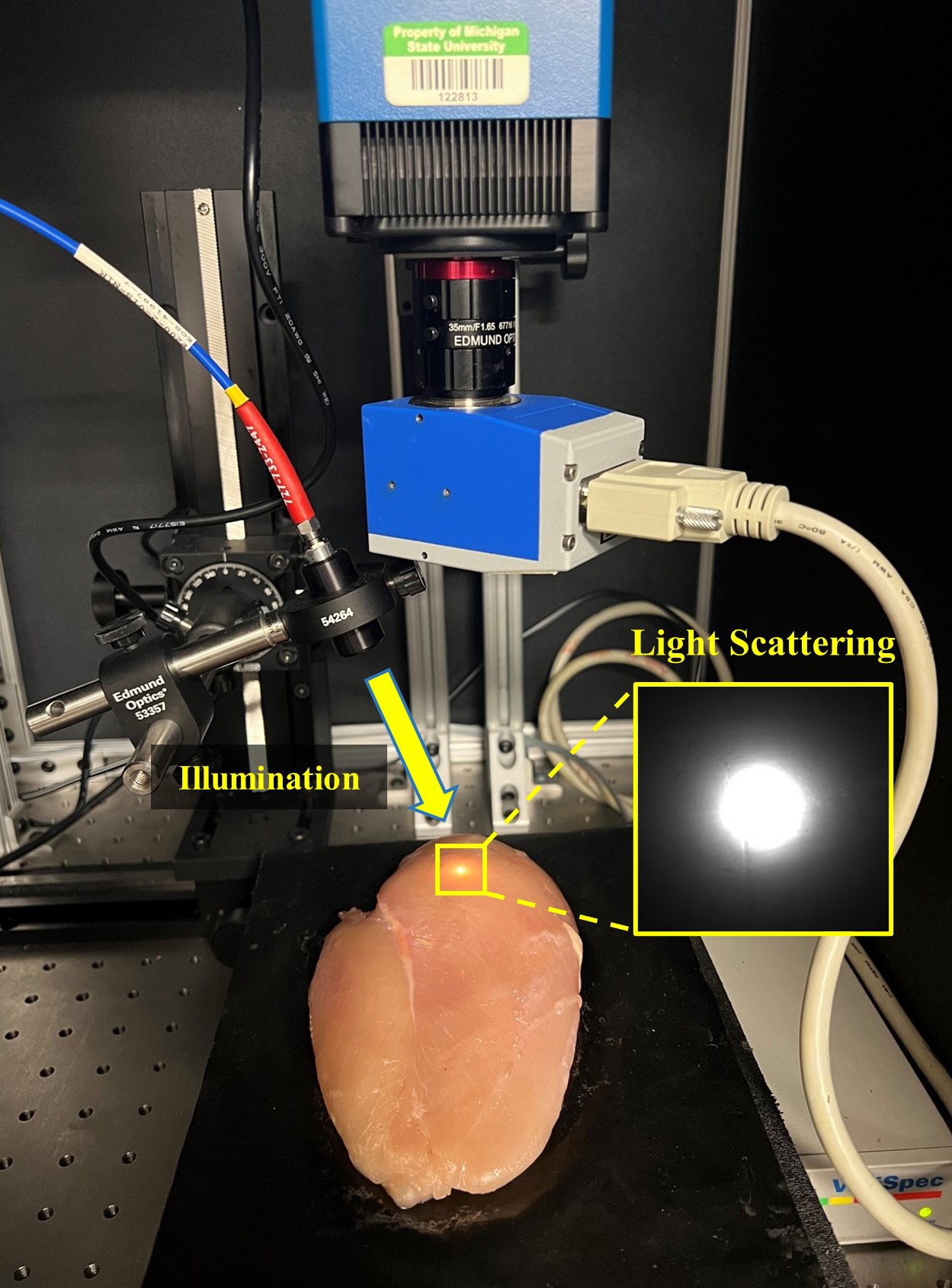
Identifying muscle myopathy in poultry is essential because the meat is condemned and pulled from the production line so it is not purchased by consumers. Consumers demand high-quality products when shopping in grocery stores and grocery stores will not buy from producers that are sending low-quality products.
Lu’s work on poultry meat imaging was formerly funded by USDA-NIFA-AFRI. He is currently partnering with the Michigan Alliance for Animal Agriculture to develop a light scattering imaging technique for woody breast assessment.